
Thus, species distribution is solely explained by the conditions and resources present in the studied sites. Niche theory 1, that predicts species are distributed according to the environmental conditions 8 and biotic interactions 9, which determine their distribution along the environmental gradient. Parallel to these advances, the development of methods that quantify the importance of spatial and environmental filters as community predictors 4, 5, 6 have been essential for interpreting the distribution patterns of species in communities 7. Understanding the distribution of biological communities along environmental and spatial gradients has had several theoretical advances such as the Niche concept 1, Neutral Theory 2, and the Meta communities synthesis 3. However, when the objectives concern conservation of biodiversity and functional diversity, rare species must be carefully assessed by other complementary methods, since they are not well represented in varpart models. Therefore, our findings suggest that studies using varpart with single sampling events that do not detect rare species can efficiently assess general distributional patterns of communities along environmental and spatial gradients. Common species are important and rare species are irrelevant for understanding the relationships among communities and environmental and spatial gradients using varpart. In all scenarios, removing up to 50% of rare species did not reduce model residuals. After the repetitions and removal of species, our hypothesis was not corroborated. We built a repetition structure, in which we gradually removed common and rare species independently. We used several environmental variables and spatial filters as varpart model predictors of fish and Zygoptera (Insecta: Odonata) communities in 109 and 141 Amazonian streams, respectively.
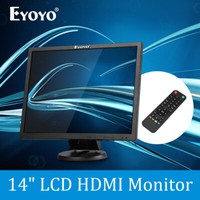
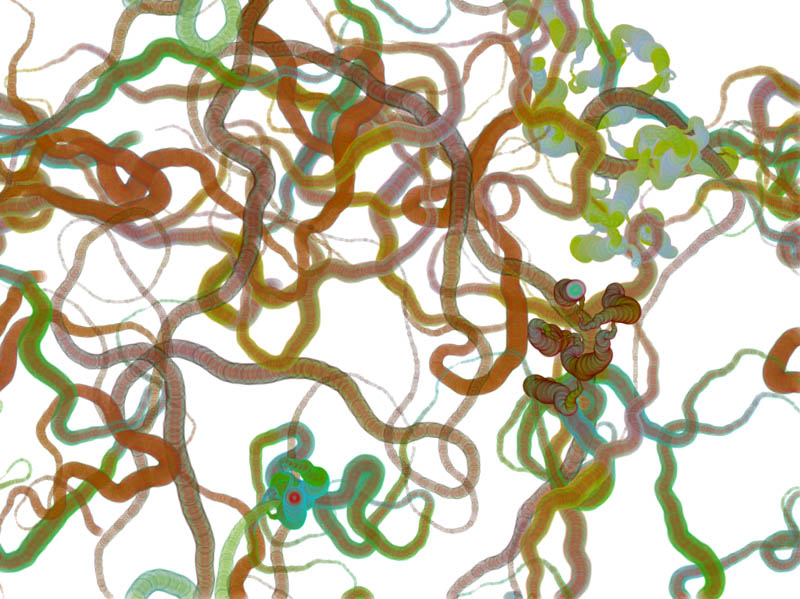
Our hypothesis is that the residual of partition the variation of community matrix (varpart) models will decrease as rare species get removed. The objective of this study is to test the relationship among communities and environmental and spatial predictors by evaluating the relative contribution of common and rare species to the explanatory power of models. It is believed that species’ intrinsic characteristics such as rarity can contribute to large residuals. However, it is common for the residuals of models investigating those relationships to be very high (> 50%). An R function to apply the SSEF testing method is provided and detailed in a tutorial.In community ecology, it is important to understand the distribution of communities along environmental and spatial gradients. This highlighted that an ISD can be properly detected providing that the spatial pattern of the filtering process is correctly captured by the sampling design of the study. However, the spatial resolution allowed by the sampling design limited the power of the method when using a fine‐scale filtering variable. The method proposed provided a correct type I error rate ( 0.9) when abundances were filtered by an environmental variable structured at broad scale. The SSEF was tested for 1000 simulated tree distributions either unrelated to the environment, or filtered by environmental variables displaying contrasting spatial structures. Ten types of tree distribution displaying contrasted aggregation properties were simulated, and their abundances were sampled in 153 regularly‐distributed 20 × 20 m quadrats. We investigated the type I error rate and statistical power of our method based on two real environmental datasets and simulations of tree distributions. To bridge the gap, we propose to test the SSEF through spatially‐constrained null models: torus‐translations, and Moran spectral randomisations. A reliable test of this fraction is therefore crucial to properly test the presence of an ISD on ecological data. the reflection of a spatially structured habitat filter on a species distribution).
#Variation partitioning environment space time rcode rscript driver
Yet, the SSEF is expected to encompass a major driver of community assembly, that is, an induced spatial dependence effect (ISD i.e. However, while these pure fractions can be tested using a classical residuals permutation procedure, no specific method has been developed to test the shared space‐environment fraction (SSEF).
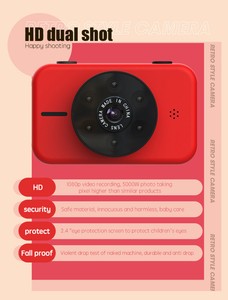
274-285 ISSN: 0030-1299 Subject: biogeography, data collection, environmental factors, habitats, models, species abundance, trees Abstract: Variation partitioning analyses combined with spatial predictors (Moran's eigenvector maps, MEM) are commonly used in ecology to test the fractions of species abundance variation purely explained by environment and space. Hardy, Thomas Drouet Source: Oikos 2019 v.128 no.2 pp. Testing and interpreting the shared space‐environment fraction in variation partitioning analyses of ecological data Author: David Bauman, Jason Vleminckx, Olivier J.
